Since the first reports of the relation between copy number variations (CNVs) and human diseases, CNV analysis has become an integral part of clinical genetics. NGS, which is routinely used in small variant detection, promises detection of CNVs as well, though with some caveats.
Here, we report a benchmark study using SEQ Platform’s CNV analysis tool, which employs the GATK gCNV pipeline, and outline the importance of the sample size and sequencing depth on the sensitivity and specificity of the analysis. As expected, read depth is the major contributor to increased sensitivity. Cohort size also has positive correlation with the sensitivity albeit to a lesser extent. Although there are diminishing returns, the higher the depth and larger the cohort, the more sensitive the CNV analysis. “Sweet spot” for the balance between cost and reliability seems to be around 150X read depth.
Copy Number Variations and Disease
First reports on Copy Number Variations (CNV) were published in 2004 by two separate groups (Iafrate et al., 2004; Sebat et al., 2004). Their findings showed that, even in healthy individuals, hundreds of kilo base pairs of DNA sequence have losses or gains in dosage compared to the reference human genome. These findings opened a new field in which the association between changes in copy numbers of various genomic regions and genetic diseases are investigated. Numerous articles connecting CNVs with various disorders such as Prader-Willi and Angelman syndromes (Redon et al., 2006), breast cancer (Peiró, Mayr, Hillemanns, Löhrs, & Diebold, 2004), and complex neurological disorders (Freeman et al., 2006; Zarrei et al., 2019) have been published since then. Currently, there are 66 CNV syndromes involved in developmental disorders and 733 disorders associated with CNVs are listed in the DECIPHER database (Firth et al., 2009). Along with SNPs, MNP and indels, CNVs have become an invaluable source of information for health care professionals for diagnosis and, in some cases, treatment.
How are CNVs detected?
Large CNVs can be detected via fluorescence in situ hybridization (FISH) or comparative genomic hybridization (CHG). Multiplex ligation-dependent probe amplification (MLPA) can also be employed for increased resolution in CNV detection. With recent advancements in data quality and wide application of next generation sequencing (NGS) techniques, detection of CNVs using the NGS data has become possible.
Limitations of CNV detection via NGS data analysis
Good quality data is essential to obtain a CNV analysis with high sensitivity and specificity. There are mainly three factors affecting the data quality:
1.Read depth: the higher the depth, the more reliable are the results
2. Size of the CNVs: the larger the CNV, the easier it is to reliably detect
3. Cohort size: the more samples in the cohort, the more sensitive and reliable are the results
In addition to these limiting factors, one should always keep in mind that a CNV analysis using NGS data should ALWAYS be performed using samples with the same kit/sequencing platform combination, and ideally all samples should be prepared in the same day, by the same person, using the same kit and run at the same machine all together in the same flow cell. Not abiding with one or more of these points may compromise the results.
In a clinical setting, we do not have any control over the size of the CNVs, and limited control over the cohort size. In large hospitals or clinics with high patient load, large cohort sizes can be obtained by accumulating patient data before the analysis. This, of course, depends on the urgency of the results and how fast the data accumulates. Read depth, on the other hand, is completely under the control of the health care professionals and/or researchers. Since the cost is always a large part of the equation, the balance between the cost and benefits obtained from a deeper sequencing should be taken into account.
There is one question pertinent to this decision:
How do the sequencing depth and the size of the cohort affect the specificity and sensitivity of the CNV analysis?
To answer this important question, we have used the ICR96 exon CNV validation series with Prof. Nazneen Rahman’s kind permission (Mahamdallie et al., 2017). This high quality data set comprises 96 independent samples prepared using the TruSight Cancer Panel and has around 600X depth, provided with matched Multiplex Ligation-dependent Probe Amplification (MLPA) results.
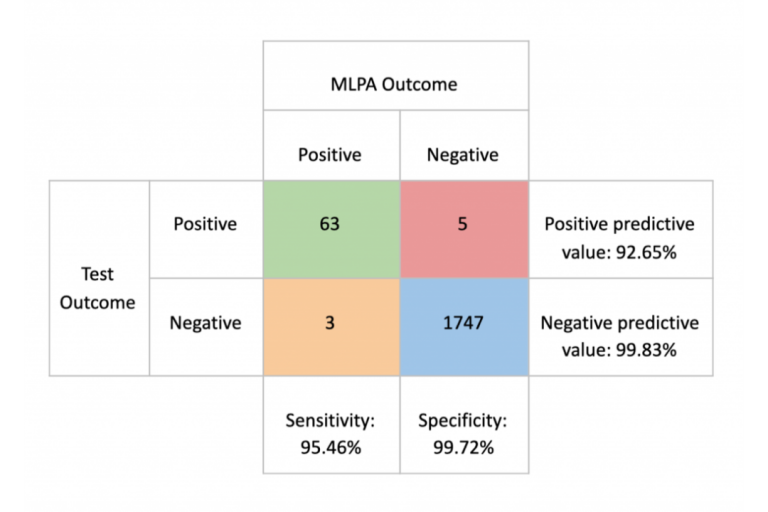
Using this data set with exceptionally high quality, the SEQ Platform’s CNV analysis, which employs the GATK gCNV pipeline (Babadi, Lee, & Smirnov, 2018), produces equally exceptional sensitivity and specificity scores
Producing patient data with similar quality to ICR96 data set may not be feasible in a clinical setting for two reasons;
1. It will be roughly 5 to 10 times more expensive than producing a WES data with widely accepted norm of 50 to 100 X depth
2. Obtaining a cohort size of 96 may take weeks or even months for small to mid-sized centers
To simulate a clinical patient and data flow, we have reduced the read depths using downsampling option in SAMtools (Li et al., 2009) to obtain ~60X and ~150X depths in addition to the original ~600X, and analyzing the data set as a whole or in groups of 8.
This analysis yielded a wide variation in the sensitivity between “high quality” and “every day scenario” samples, ranging from 95.45% to 42.42%. Specificity, on the other hand, remained above 99.6% in all scenarios.
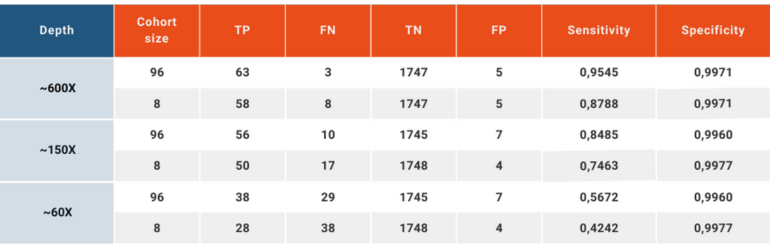
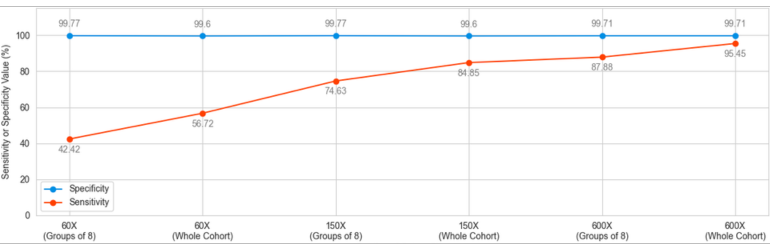
Conclusion
CNV analysis using NGS data makes use of the already available data produced to analyze small variations carried by the patient and adds another layer of invaluable information without creating additional cost. This analysis, however, has inherent limitations. The size of the sample pool, the average read depth, coverage values, length of the CNV and the conditions under which the NGS libraries are prepared and run have effects of various degrees on the quality and reliability of the obtained results. SEQ Platform’s CNV analysis produces exceptionally high sensitivity and specificity scores when the raw data has high read depth and the size of the cohort is high. When the same analysis is performed using a data set simulating the NGS data obtained in day-to-day operations in today’s hospitals and clinics, the sensitivity is greatly affected going from 95.45% in cohort of 96 samples with ~600X depth down to 42.42% in a cohort analyzed in chunks of 8 and has ~60X average read depth. In both extreme cases, however, the specificity remains above 99.6%, meaning that although the ability of the CNV analysis to detect the variations in the sample is affected by read depth and the number of samples included in the same analysis, reported positive variants are largely true. These values are in agreement with previous reports (Babadi, Lee, & Smirnov, 2018; Zhao, Liu, Yuan, Gao, & Duan, 2020). Although CNV analysis using NGS data provides valuable additional information, health care practitioners, researchers and analysts should always consider the relation between the sample quality and reliability of results.
References
Babadi, M., Lee, S. K., & Smirnov, A. N. (2018). GATK gCNV: accurate germline copy-number variant discovery from sequencing read-depth data. The International Conference on Probabilistic Programming (PROBPROG).
Firth, H. V, Richards, S. M., Bevan, A. P., Clayton, S., Corpas, M., Rajan, D., … Carter, N. P. (2009). DECIPHER: Database of Chromosomal Imbalance and Phenotype in Humans Using Ensembl Resources. American Journal of Human Genetics, 84(4), 524–533. https://doi.org/10.1016/j.ajhg.2009.03.010
Freeman, J. L., Perry, G. H., Feuk, L., Redon, R., McCarroll, S. A., Altshuler, D. M., … Lee, C. (2006). Copy number variation: new insights in genome diversity. Genome Research, 16(8), 949–961. https://doi.org/10.1101/gr.3677206
Iafrate, A. J., Feuk, L., Rivera, M. N., Listewnik, M. L., Donahoe, P. K., Qi, Y., … Lee, C. (2004). Detection of large-scale variation in the human genome. Nature Genetics, 36(9), 949–951. https://doi.org/10.1038/ng1416
Li, H., Handsaker, B., Wysoker, A., Fennell, T., Ruan, J., Homer, N., … Durbin, R. (2009). The Sequence Alignment/Map format and SAMtools. Bioinformatics (Oxford, England), 25(16), 2078–2079. https://doi.org/10.1093/bioinformatics/btp352
Mahamdallie, S., Ruark, E., Yost, S., Ramsay, E., Uddin, I., Wylie, H., … Rahman, N. (2017). The ICR96 exon CNV validation series: a resource for orthogonal assessment of exon CNV calling in NGS data. Wellcome Open Research, 2, 35. https://doi.org/10.12688/wellcomeopenres.11689.1
Peiró, G., Mayr, D., Hillemanns, P., Löhrs, U., & Diebold, J. (2004). Analysis of HER-2/neu amplification in endometrial carcinoma by chromogenic in situ hybridization. Correlation with fluorescence in situ hybridization, HER-2/neu, p53 and Ki-67 protein expression, and outcome. Modern Pathology, 17(3), 277–287. https://doi.org/10.1038/modpathol.3800006
Redon, R., Ishikawa, S., Fitch, K. R., Feuk, L., Perry, G. H., Andrews, T. D., … Hurles, M. E. (2006). Global variation in copy number in the human genome. Nature, 444(7118), 444–454. https://doi.org/10.1038/nature05329
Sebat, J., Lakshmi, B., Troge, J., Alexander, J., Young, J., Lundin, P., … Wigler, M. (2004). Large-Scale Copy Number Polymorphism in the Human Genome. Science, 305(5683), 525 LP – 528. https://doi.org/10.1126/science.1098918
Zarrei, M., Burton, C. L., Engchuan, W., Young, E. J., Higginbotham, E. J., MacDonald, J. R., … Scherer, S. W. (2019). A large data resource of genomic copy number variation across neurodevelopmental disorders. Npj Genomic Medicine, 4(1), 26. https://doi.org/10.1038/s41525-019-0098-3
Zhao, L., Liu, H., Yuan, X., Gao, K., & Duan, J. (2020). Comparative study of whole exome sequencing-based copy number variation detection tools. BMC Bioinformatics, 21(1), 97. https://doi.org/10.1186/s12859-020-3421-1